From Mind to Model: Stable Diffusion as a Bridge Between Idea and Visual Representation
Introduction
ATI Project’s experience in integrating Artificial Intelligence (AI) into the design process continues to evolve with the use of various platforms that enable greater speed and clarity in the early stages of the creative process. In the previous article, A new ally sits at our table: AI, we explored the role of Midjourney in visualizing and evaluating design concepts, highlighting how this technology allows for rapid exploration of different ideas and solutions. In this article, we will focus on the second phase of the concept development, analyzing the use of additional image generation tools and their impact on refining the design process.
Stable Diffusion and Latent Diffusion Models
Midjourney is just one of the various tools available in the generative AI market. Among the most important and useful is Stable Diffusion: an AI that uses a technique called “generative diffusion” to create coherent, high-quality images from textual descriptions (text-to-image) or to enhance and modify existing images (image-to-image).
But how does it work exactly?
Diffusion models are a type of generative models that learn how to manipulate images through two complementary phases: adding noise and then removing it.
In the first stage, known as noising, the model learns to progressively add noise to an image. This process, which gradually distorts the visual details, is essential for training the model to understand how noise patterns develop and spread in images.
Once the model has acquired this skill, it is trained to do the opposite: through the denoising process, the model removes the noise step by step, returning an image that becomes clearer and more defined. With each step, the image progressively approaches its original form, with greater detail and precision.
The final quality of the image depends on the model’s ability to recover the visual information hidden by the noise, further refining the level of detail and accuracy at each step.
To guide this process, the diffusion model uses a system developed by OpenAI called CLIP (Contrastive Language-Image Pretraining), which converts the textual description provided by the user into a numerical representation, thus steering the denoising process and allowing the AI to refine the image. This approach ensures that the final result is consistent with the description provided.
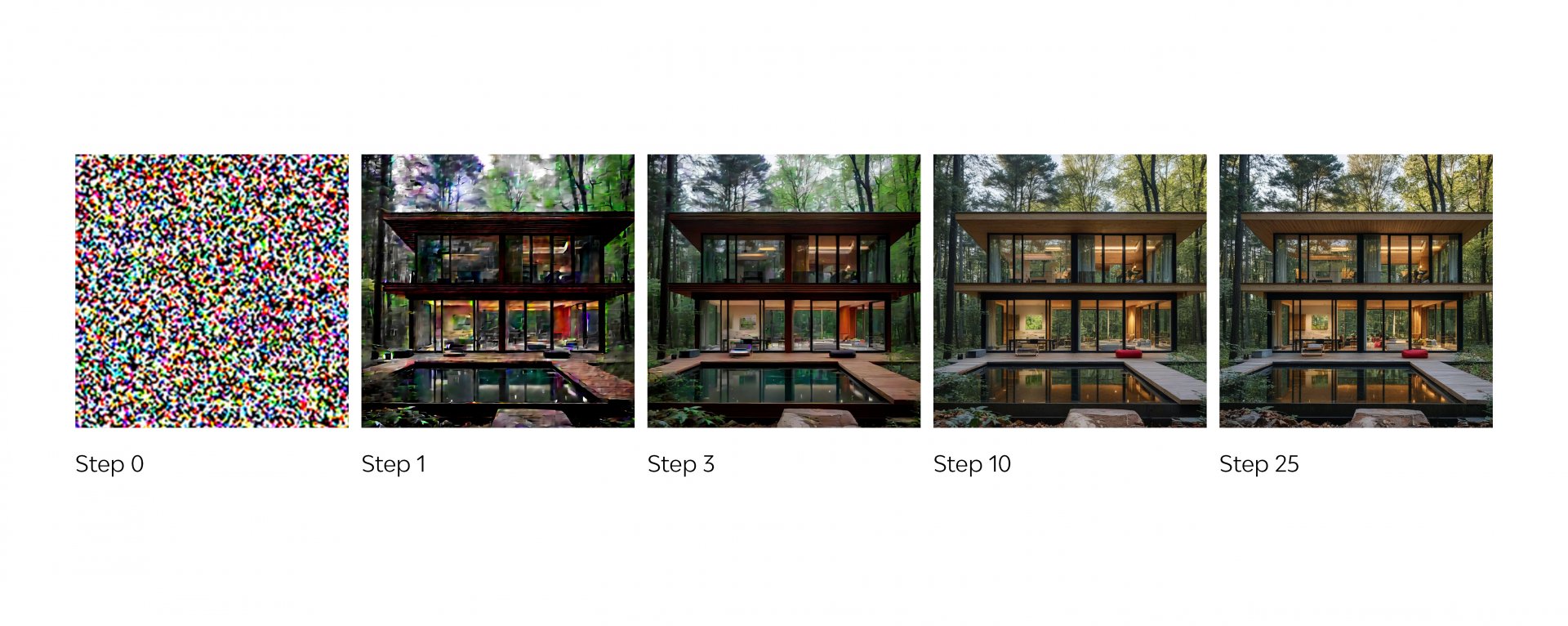
However, working directly on images in a visible space made of pixels would require a huge amount of computational resources. For this reason, Stable Diffusion uses a latent space, which is a compressed and abstract representation of the image. In this space, processing is more efficient, as the data is reduced without losing the essential information needed to generate a high-quality image.
This process is made possible by a system called encoder-decoder. The encoder takes the image from the original space and transforms it into a representation in the latent space. In the case of Stable Diffusion, the encoder is a VAE (Variational Autoencoder), which creates continuous and structured representations in this space. Once the image has been compressed into the latent space, the decoder comes into play, performing the reverse operation, bringing the latent representation back to the original space and generating a detailed, high-resolution image.
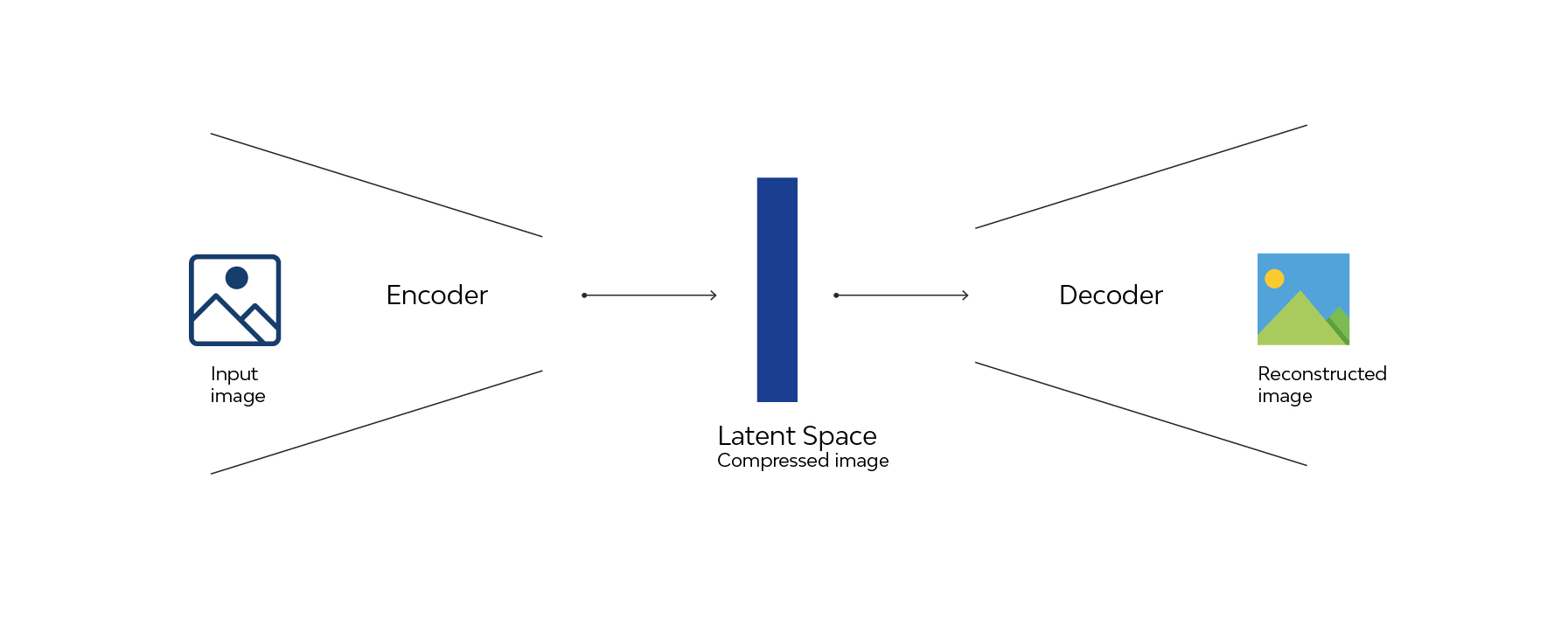
As already mentioned, the two main features of Stable Diffusion are text-to-image and image-to-image. In the first case, the system generates an image from a textual prompt, while in the second, both a prompt and a starting image are used to achieve a visually similar result.
Stable Diffusion also offers the possibility to make changes to images through inpainting in the image-to-image section. This feature allows you to create masks on images, to which a prompt is associated in order to add details or modify portions of the image. Finally, it is possible to create videos through text-to-video or video-to-video, further expanding the creative possibilities.
Influencing the Output: The Importance of Parameters in ControlNet
To expand the possibilities offered by Stable Diffusion, there is ControlNet, an advanced technology designed to provide precise, conditional control during image generation.
ControlNet is based on the idea of guiding the latent diffusion process using structured inputs such as reference images, depth maps, contours, poses, or other additional information. This approach allows for maintaining the creative flexibility of the model while adhering to specific conditions.
The operation of ControlNet involves a process that integrates a parallel network (control network) into the backbone of the Stable Diffusion model. The backbone is the pre-trained neural model responsible for image generation, which is ‘frozen’ so it is not modified while the ControlNet influences the generation path to enforce strict constraints. The separately trained ControlNet network receives structured input data, such as contour maps (e.g., Canny or Lineart), depth maps, or other specific information. This data is processed within the latent space, and the generation path influenced by the ControlNet ensures that the input data is maintained during the denoising process.
The result is a final image that not only gets refined but remains faithful to the constraints imposed by the initial input, ensuring that the generation adheres to the requested specifications. ControlNet thus provides greater precision in controlling details, representing a powerful tool for artists and designers who want to integrate AI into their creative workflows.
ControlNet, therefore, emerges as a truly revolutionary extension, expanding the versatility of Stable Diffusion by offering new opportunities for customization and fine-tuning of the generated images.
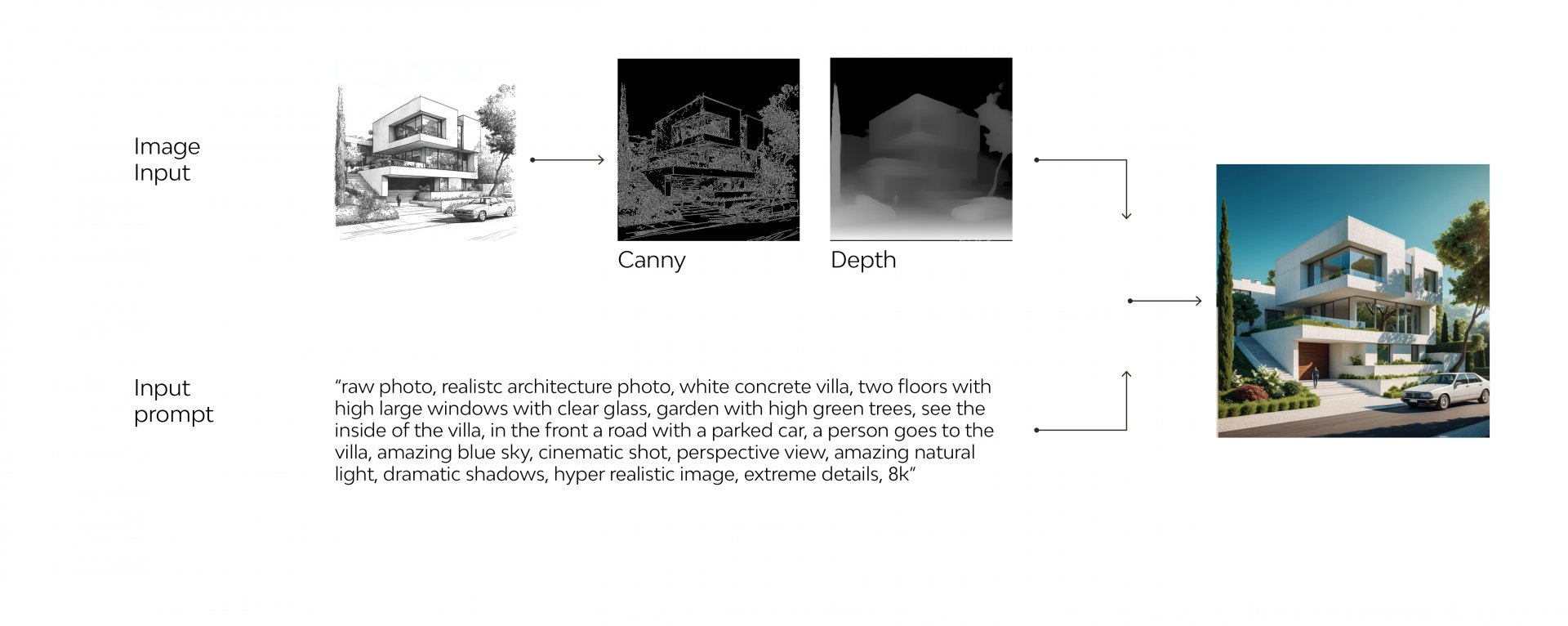
Case Study: The North Tower of Pisa
Returning to the case study discussed in the previous article, we now move on to the second step of the workflow, which requires greater control over the image to maintain accuracy in the volume and characteristics of the project.
We will focus on one of the options proposed by the matrix generated with Midjourney, which was not selected as the final design solution but offers the opportunity to explore different steps of interaction with AI. The concept under analysis features an envelope characterized by vertically varying elements, the development of which requires advanced tools for more accurate image generation.
To this end, we chose to use Comfy UI, an advanced user interface for managing AI models based on Stable Diffusion. Comfy UI provides a modular and highly customizable environment, with visual programming that allows the creation of complex workflows by combining visual nodes. Thanks to its flexibility, the interface can be adapted to different needs. In our case, two main workflows were used: image/sketch to render and real-time generation from 3D Revit and Speckle models.
Below is a description of the main steps in the process:
Step 1: Image/sketch to Render
As the first step, a base image of the Tower in the form of a sketch was used, to which various vertical slat solutions were added to the façade. At this stage, the entire building with the integrated solution can be visualized.
The generation of an image faithful to the sketch, as well as for other types of visual input, was achieved using the ControlNet extension. This is particularly useful in contexts where one starts with a simple schematic or floor plan to obtain complex and customized renderings, while ensuring consistency with the desired details.
To increase control over the appearance of the image, IPadapter was also used, which helps guide the style of the image. IPadapter is based on an adaptive network that provides additional inputs to the model, influencing the final result with specific data such as reference images, styles, or textures. In this case, the reference image used is the one generated by Midjourney.
Next, we move on to the following phase: creating a detailed 3D model.
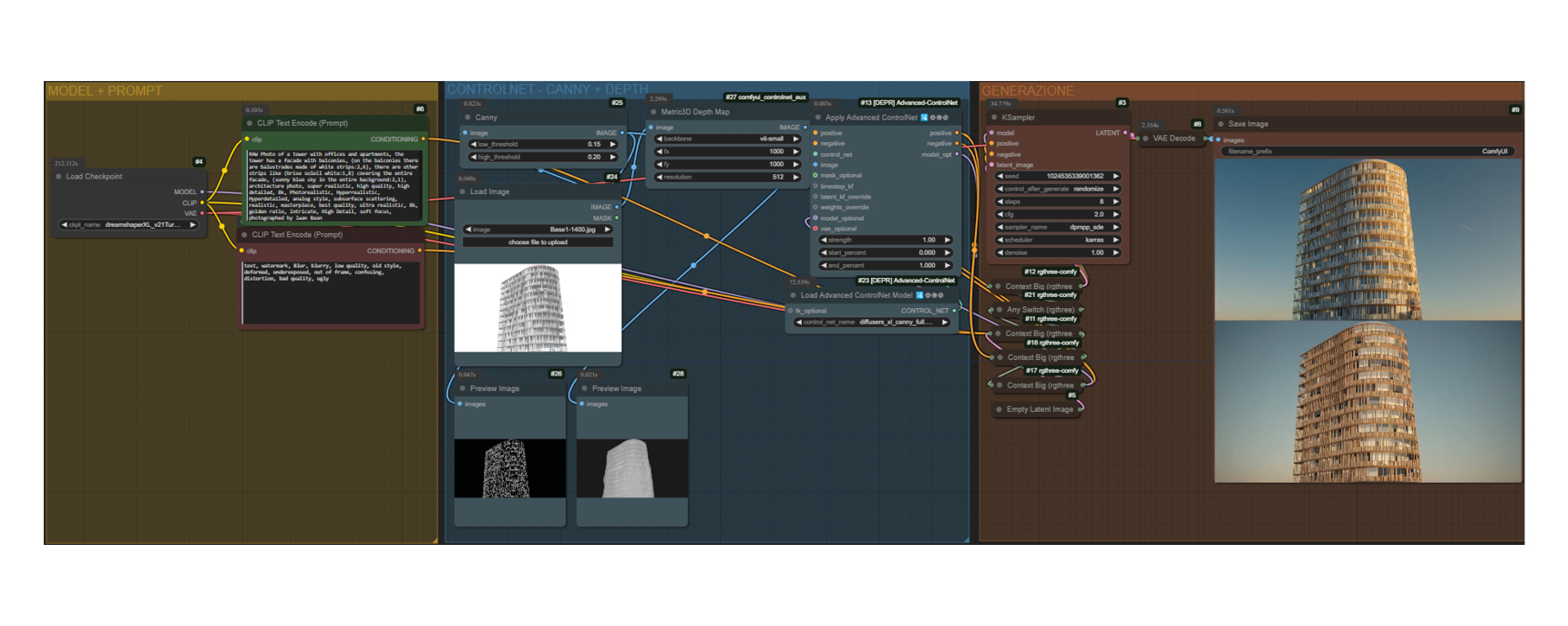
Step 2: Real-Time Generation from a 3D Model via Revit and Speckle
With the creation of a 3D model, before moving on to the final phase, Grasshopper is used to facilitate the arrangement of vertical elements on the façade. This tool allows precise management of dimensions, heights, distances, and other parameters, optimizing the process and helping achieve the most suitable solution in a short amount of time.
The model is then imported into Speckle, a program that facilitates the import and export of 3D data, allowing for more precise texture application and simplifying complex tasks such as UV projection and physically accurate material management.
Thanks to specific ‘nodes’ within Comfy UI, it is possible to link the visualization of the model in Speckle and use this connection to generate real-time images. These images adapt to the user’s movements in 3D, thus offering the ability to view real-time renders of different views and solutions of the building.
Conclusion
The generation of images and videos through AI is experiencing continuous expansion, with updates and new models released every month that open up new possibilities, improving control and the speed of final outputs in increasingly surprising ways. As highlighted in this article and the previous one, this methodology not only accelerates decision-making processes but also elevates the quality of the final result, allowing designers and project teams to visualize in real-time the impact of their choices.
The integration of AI-based workflows thus proves to be a crucial element for innovation in the architectural field, combining creativity, technology, and precision at every stage of the project.
Explore the 3D model
Video
#StableDiffusion #MidJourney#ControlNet #AIInDesign #InnovativeDesign #DigitalDesign #CreativeProcess #ImageGeneration #LatentDiffusion #ArchitecturalRendering #ConceptDevelopment #AdvancedDesign #AIForArchitecture #AIForCreativeWorkflows #AI #ImageToImage
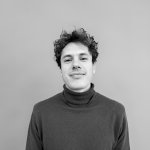