Machine Learning application on Life Cycle Assessment
The Value of Design Data in Sustainable Architecture
Introduction
Modern architecture stands at a crossroads, where the need to build sustainably is hampered by traditional design methodologies. In this context, the integration of Machine Learning (ML) into Life Cycle Assessment (LCA) emerges as a revolution, providing a fresh perspective on handling design data.
Data collected from past projects, though rich in information, often go unused or undervalued due to limitations in traditional analysis methods. Machine Learning, with its ability to process and analyze vast amounts of data, offers a solution to transform these datasets into valuable insights.
The collaborative project with Scuola Normale Superiore and the CNR
A concrete example of this innovation is the proof-of-concept (PoC) developed by ATI Project within the European project SoBigData for Industry: Challange Us 2023 in collaboration with Scuola Normale Superiore and the Consiglio Nazionale delle Ricerche (CNR) of Pisa.
This project involved the use of Machine Learning to analyze design data and provide predictive assessments of the environmental impact of buildings.
Being able to make these assessments in the early stages of design is crucial for minimizing carbon footprint and optimizing resource utilization. Therefore, the analysis of Life Cycle Assessment is essential, as it can evaluate the environmental impact of a building in all phases of its life cycle—the production and construction phase of materials, the management and maintenance phase, and the end-of-life phase—based on their impact on carbon emissions.
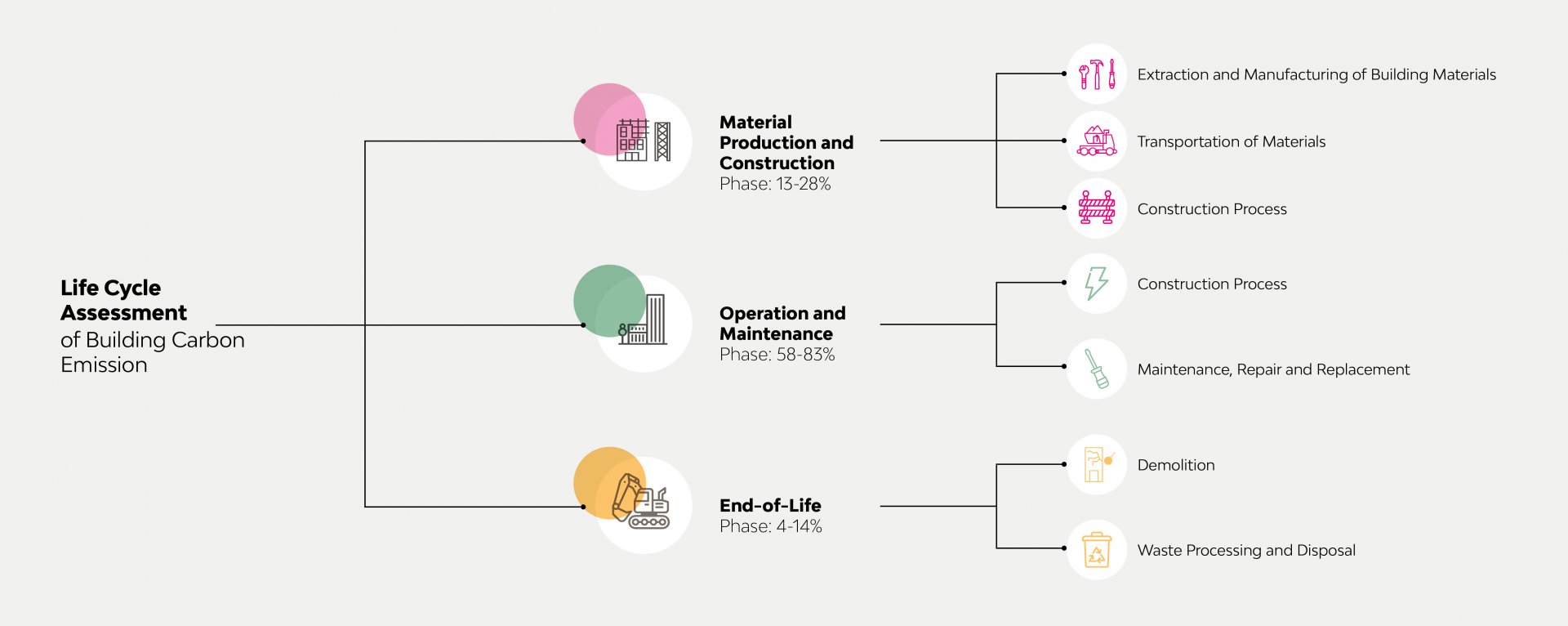
The management phase is particularly critical as it accounts for half of the total CO2 emissions of the building. The impact of this phase is largely driven by its energy consumption, a data point that typically requires extensive information collection not always available in the early design stages.
Thanks to the synergistic collaboration that led to the development of the PoC, it became possible to create Machine Learning models capable of predicting the energy efficiency classes of future constructions in the initial design phases. Data from ATI Project’s server design documents were extracted, indexed, and subsequently used to train the model in extracting input features for training. The data collection process of the study begins with a thorough analysis of the energy certification of buildings, known as ‘Legge 10’, a mandatory national standard for all completed construction projects. These documents contain comprehensive data on various aspects such as energy consumption, the use of renewable energy sources, and building system efficiency metrics. Additionally, they provide geographic information, including climatic conditions and location.
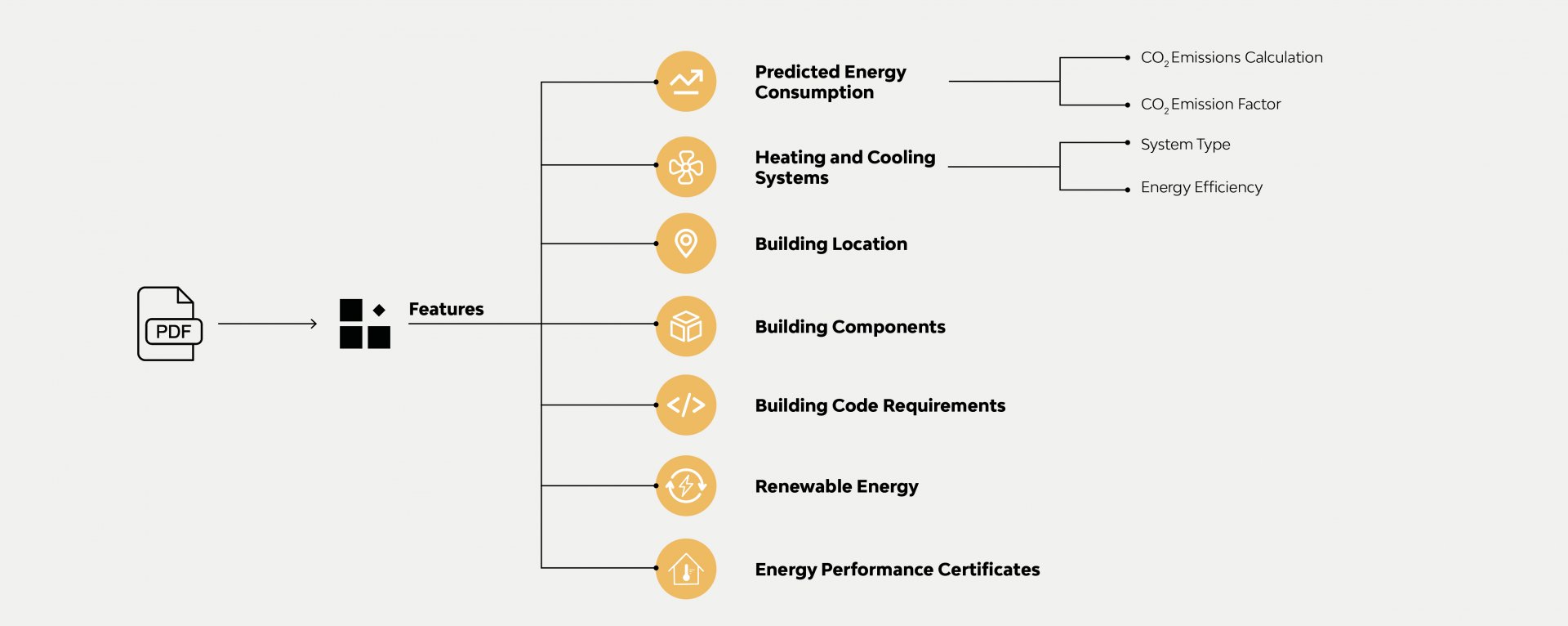
However, despite the wealth of information and data regarding the energy aspects of the building, these documents do not contain all the necessary input features to develop the Machine Learning model for LCA analysis. The next step involves the integration of additional data from Industry Foundation Classes (IFC) files. IFC files are a globally recognized standard for the sharing and exchange of construction and facility management data, providing a more comprehensive view of a building. This open BIM format allows us to identify all the significant features for a Machine Learning model, not only for the purpose of the PoC but also for all the variables involved in LCA methods.
The impact and the potential of Machine Learning
The integration of Machine Learning has shown significant potential, not only in predicting energy efficiency but also in optimizing material usage and reducing the overall ecological impact. This approach provides architects and designers the opportunity to explore and evaluate different design options in a more informed and conscious manner, contributing significantly to global sustainability goals.
“The generation of new knowledge from existing knowledge, often achieved through problem-solving, is a key component of the creative process”. Arthur I. Miller
This approach emphasizes that creativity often does not emerge within an isolated context; instead, it is an iterative and dynamic process that relies on what is already known. Through problem-solving, individuals can connect and rework existing knowledge to generate new ideas, discoveries, or solutions, thereby contributing to the advancement of knowledge in a specific field or across various interdisciplinary domains
Toward a sustainable future in architecture
The collaboration between data science and BIM design opens the doors to a future where sustainability is no longer just a goal to pursue but an integrated reality in the design process. The use of Machine Learning to predict the necessary data in the early stages of a project represents a qualitative leap in the approach to sustainable construction. This new paradigm not only enhances the efficiency and sustainability of buildings but also fosters greater ecological awareness in the construction industry.
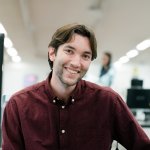